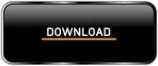
Self-supervised learning on graphs: Deep insights and new direction. Wei Jin, Tyler Derr, Haochen Liu, Yiqi Wang, Suhang Wang, Zitao Liu, and Jiliang Tang.In KDD '21: The 27th ACM SIGKDD Conference on Knowledge Discovery and Data Mining. Cross-Network Learning with Partially Aligned Graph Convolutional Networks. Advances in Neural Information Processing Systems 33 (2020), 5862-5874. In KDD '20: The 26th ACM SIGKDD Conference on Knowledge Discovery and Data Mining. GPT-GNN: Generative Pre-Training of Graph Neural Networks. Ziniu Hu, Yuxiao Dong, Kuansan Wang, Kai-Wei Chang, and Yizhou Sun.In 8th International Conference on Learning Representations, ICLR. Strategies for Pre-training Graph Neural Networks. Weihua Hu, Bowen Liu, Joseph Gomes, Marinka Zitnik, Percy Liang, Vijay S.Advances in neural information processing systems 33, 22118-22133. Open Graph Benchmark: Datasets for Machine Learning on Graphs. Weihua Hu, Matthias Fey, Marinka Zitnik, Yuxiao Dong, Hongyu Ren, Bowen Liu, Michele Catasta, and Jure Leskovec.In Advances in Neural Information Processing Systems 30: Annual Conference on Neural Information Processing Systems. Inductive Representation Learning on Large Graphs. Hamilton, Zhitao Ying, and Jure Leskovec. Kai Guo, Kaixiong Zhou, Xia Hu, Yu Li, Yi Chang, and Xin Wang.In International conference on machine learning. Neural message passing for quantum chemistry. Justin Gilmer, Samuel S Schoenholz, Patrick F Riley, Oriol Vinyals, and George E Dahl.In Proceedings of the 24th ACM SIGKDD International Conference on Knowledge Discovery & Data Mining, KDD 2018. Large-Scale Learnable Graph Convolutional Networks. Hongyang Gao, Zhengyang Wang, and Shuiwang Ji.IEEE Transactions on Pattern Analysis and Machine Intelligence (2022). Bag of Tricks for Training Deeper Graph Neural Networks: A Comprehensive Benchmark Study. Tianlong Chen, Kaixiong Zhou, Keyu Duan, Wenqing Zheng, Peihao Wang, Xia Hu, and Zhangyang Wang.In International Conference on Machine Learning. Simple and deep graph convolutional networks. Ming Chen, Zhewei Wei, Zengfeng Huang, Bolin Ding, and Yaliang Li.In 6th International Conference on Learning Representations, ICLR. Deep Gaussian Embedding of Graphs: Unsupervised Inductive Learning via Ranking. Aleksandar Bojchevski and Stephan Günnemann.The extensive experiments on eight benchmark datasets demonstrate the superiority of GPPT, delivering an average improvement of 4.29% in few-shot graph analysis and accelerating the model convergence up to 4.32X. Therefore, the pre-trained GNNs could be applied without tedious fine-tuning to evaluate the linking probability of token pair, and produce the node classification decision. The token pair is consisted of candidate label class and node entity. Based on the pre-trained model, we propose the graph prompting function to modify the standalone node into a token pair, and reformulate the downstream node classification looking the same as edge prediction. Specifically, we first adopt the masked edge prediction, the most simplest and popular pretext task, to pre-train GNNs. To bridge the task gap, we propose a novel transfer learning paradigm to generalize GNNs, namely graph pre-training and prompt tuning (GPPT). Even worse, the naive pre-training strategy usually deteriorates the downstream task, and damages the reliability of transfer learning in graph data. This significant gap often requires costly fine-tuning for adapting the pre-trained model to downstream problem, which prevents the efficient elicitation of pre-trained knowledge and then results in poor results. However, they rarely notice the inherent training objective gap between the pretext and downstream tasks. Recently, many efforts have been paid to design the self-supervised pretext tasks, and encode the universal graph knowledge among the various applications. An effective solution is to apply the transfer learning in graph: using easily accessible information to pre-train GNNs, and fine-tuning them to optimize the downstream task with only a few labels. Despite the promising representation learning of graph neural networks (GNNs), the supervised training of GNNs notoriously requires large amounts of labeled data from each application.
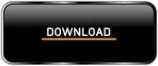